Layoffs in Responsible AI Teams
Some inferences from layoffs in responsible AI teams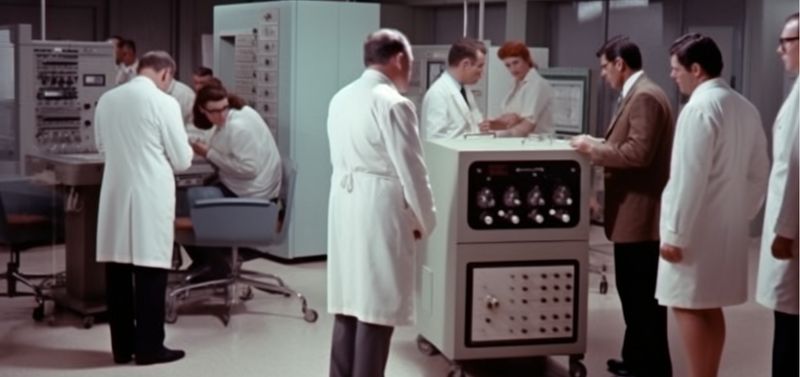
Wendy Grossman asks “what about all those AI ethics teams that Silicon Valley companies are disbanding? Just in the last few weeks, these teams have been axed or cut at Microsoft and Twitch...” and I have a theory.
My theory is informed by a conversation that I had with Michael Howard, maybe 20 years ago. I was, at the time, a big proponent of code reviews, and I asked about Microsoft’s practices. He said, “oh, they don’t scale, we don’t do [require] things that don't scale.” (Or something like that. It was a long time ago.) After I joined the SDL team, and we started working together, I saw the tremendous focus that the team had on bugs. (My first day on the job included an all-hands, and I saw GeorgeSt present how many bugs the Secure Windows Initiative had managed through the Vista process.)
Bugs, tickets, stories and the like are all intended as actionable elements of software development. ‘Stop and reflect’ doesn’t fit that mold. One of the advantages we get moving from 'evil brainstorming' to the Four Question Frame for threat modeling is that it lets us define specific tasks and relate them to other work.
But it seems ‘stop and reflect’ is often a key part of what responsible AI researchers advocate. One possible takeaway is such a focus would be helpful for ethical AI teams. I suspect that there are extreme ‘impedance mismatches’ between software developers and people who get PhDs in ethical AI. It’s also possible to think that such a focus would be putting a bandaid on when we need a tourniquet. The DAIR Institute’s response to the AI Pause letter calls for transparency and accountability, enforced by regulation: “but organizations building these systems should also be required to document and disclose the training data and model architectures. The onus of creating tools that are safe to use should be on the companies that build and deploy generative systems, which means that builders of these systems should be made accountable for the outputs produced by their products.”
Since we do not have such regulation, and (as far as I know) no AI lab has actually stepped up to announce that they’re pausing, perhaps a short-term focus on short-termism would be helpful. What makes for a stop-ship bug in an ML model? Perhaps I’ve missed the work that provides such definitions.
A crucial lesson is the value that actionability provides. For example, one laid off person said: “People would look at the principles coming out of the office of responsible AI and say, ‘I don’t know how this applies,’” (The Verge). That same article characterizes the work as “the team has been working to identify risks posed by Microsoft’s adoption of OpenAI’s technology throughout its suite of products.” Again, a lesson from how we’ve come to define threat modeling is that we cannot stop with the question “what can go wrong,” but we must get to “what are we going to do about it?”
Many people are arguing that risks of AI are so monumental that we shouldn’t try to work within the structures which exist within companies, but regulate. There are complexities there, including that regulation mainly impacts ethical players in the market. But perhaps we can learn from similar efforts and make headway while we wait for regulatory action.
Image: Midjourney, “a large team of scientists in lab coats teaching a mainframe computer about ethical behavior. Scientists, some with clipboards, can be seen displaying deep concern and are in intense discussion in small groups 1960s cinematic, hyper-realistic, nasa. --v 5 --ar 16:9” I chose to leave the all-white output, and appreciated the various AI oddities.
Edited based on a comment from Michael that he probably said something about not treating code reviews as mandatory.